P51 - Tuning Atmospheric Turbulence Parameters with Machine Learning Surrogates
Description
Parameterizations of subgrid-scale (SGS) processes, like cloud microphysics, radiation, or turbulence, cause considerable uncertainty in numerical climate and weather models at various spatiotemporal scales. Tuning the involved model parameters is challenging, given the immense computational cost of model evaluations, and the reliance on empirical judgement. The transition of numerical weather prediction to convective scales (spatial resolutions of hundreds of meters) is accompanied by new data assimilation methods including parameter estimation. However, their performance is limited by either simplified model representations or repeated model evaluations. For more objective calibration, using iterative Bayesian methods (MCMC algorithms), fast and accurate model surrogates are needed. The recent advances of data-driven full-model emulators, that avoid explicit SGS modeling, motivates the extension of such models to capture the effects of SGS parameters. Here, we focus on turbulence parameterizations in large-eddy simulations (LES) with resolutions of tens of meters. In order to accurately represent turbulence, emulators of LES simulations have to capture both the variability of the resolved turbulent motion (probabilistic/ensemble forecast) and its mean state. To this end, we compare extensions of deterministic forward emulators, such as neural operators, for probabilistic forecasting of idealized atmospheric test cases, in order to assist model calibration.
Presenter(s)
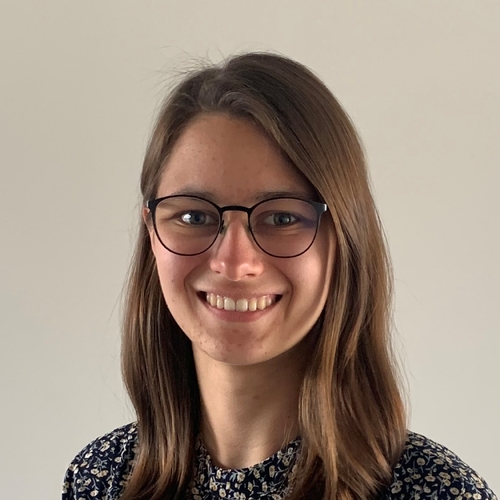
Presenter
Dana Grund is a PhD student at ETH Zürich. Based on her studies in applied mathematics in Münster (Germany), Madrid, and at ETH Zürich, she seeks to put results on computational modelling of PDEs into practice in geophysical applications. She is particularly interested in exploring the use of machine learning for classical tasks of numerical modelling. Dana is part of both the atmospheric circulation group (Prof. Schemm) and the computational and applied math laboratory (Prof. Mishra) at ETH Zürich. She is working with Large eddy simulations, with a focus on parameter estimation and emulation of numerical solvers with neural operators.